Predictive maintenance
By implementing a predictive maintenance strategy, you gain actionable data for your maintenance and asset management approach. Industrial operators must adopt predictive maintenance to anticipate anomalies and reduce costs.
Anticipate anomalies before they occur.
End unplanned downtime
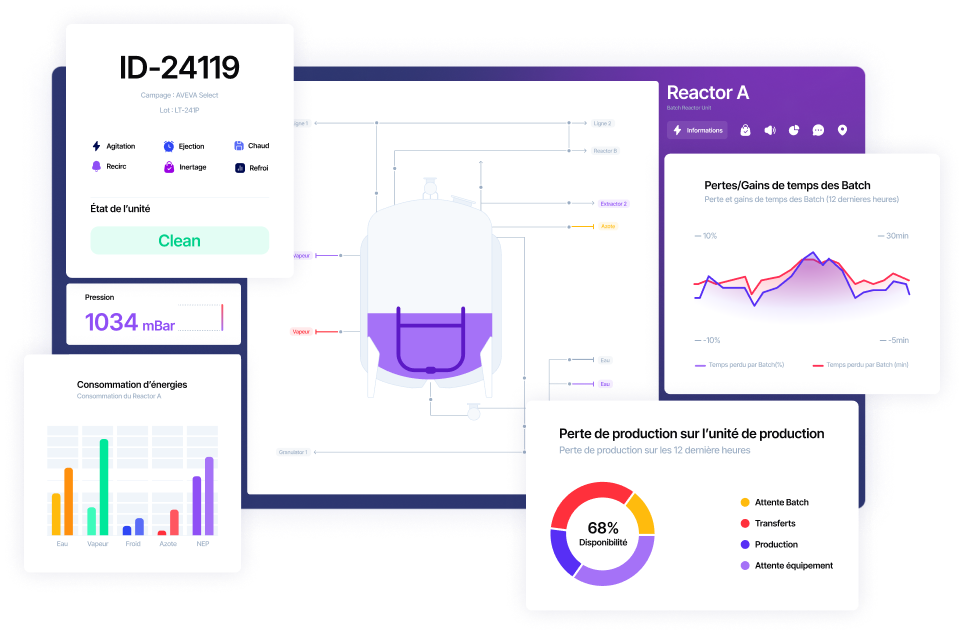
Improving plant and asset efficiency with predictive maintenance
Up to 50%
Reduction in time required for maintenance planning
Up to 20%
Increase in equipment uptime
Up to 10%
Reduction in overall maintenance costs
prev
next
Presentation
Predictive maintenance to anticipate anomalies and reduce costs.
Currently, you are likely applying a reactive maintenance approach, which allows you to intervene only when equipment fails. This method is costly and results in downtime that affects productivity. With AVEVA software, we offer an approach based on condition-based monitoring, meaning that we collect data while the machines are operating. Today, we are advancing further with predictive maintenance, which incorporates model-based anomaly detection. This strategy utilizes the continuous online collection of sensor data, enhanced by data analytics and AI, to predict machine reliability more accurately.
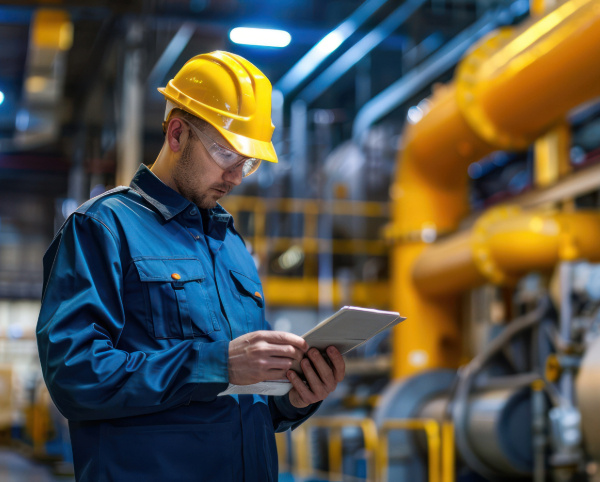
End Unplanned Downtime
AVEVA Predictive Analytics software has proven instrumental in preventing unplanned outages across industries. For example, Duke Energy saved over 34 million€ with early warnings, and SCG Chemicals increased plant reliability to 100%. The global market for predictive analytics is expected to grow significantly, driven by advancements in AI and machine learning. Companies must consider ROI, hardware compatibility, and ease of deployment when choosing predictive analytics tools. AVEVA's solutions, including AVEVA Predictive Analytics and AVEVA PI Data Infrastructure, provide advanced alert notifications, fault diagnostics, and prescriptive actions, improving operational efficiency and reducing OPEX by up to 20%. Notably, Mitsubishi Power utilized these tools to enhance operational awareness and prevent unplanned shutdowns.
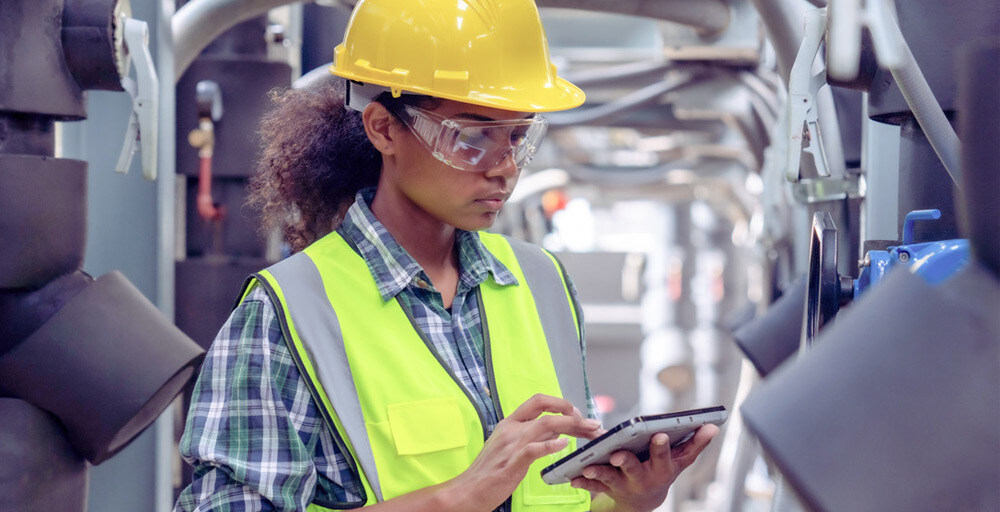
The 4 steps to implement your predictive maintenance strategy
Establish an Operations-Data Infrastructure
Use an enterprise operations-data infrastructure like the PI System to capture and transform real-time data from sensors and equipment into valuable insights. This enhances asset reliability, process efficiency, and management of energy and resources, while also improving environmental, health, and safety outcomes, product quality, and performance reporting.
Enhance and contextualize data
Ensure data collection is complemented by effective enhancement to transform it into valuable information. Providing context to data, like discerning whether a machine stoppage is due to a malfunction or an emergency stop, adds meaning. This helps analysts determine if data points indicate potential failures or are unrelated events. The PI System helps by offering contextualized data, enabling smarter operations.
Implement condition-based maintenance
Predictive maintenance involves adopting a condition-based maintenance (CBM) approach using contextualized data. This approach identifies conditions leading to asset failure and automates maintenance based on the asset's current state. For example, rising bearing temperatures can indicate impending failure, allowing real-time alerts for timely repairs. The PI System can enhance CBM with basic analytics, making maintenance strategies more predictive by recognizing performance patterns. Known failure patterns from reliability analysis can be implemented in a real-time data infrastructure.
Implement predictive maintenance 4.0
Implement predictive maintenance 4.0 (PdM 4.0). The PI System, combined with advanced analytics and pattern recognition tools, provides real-time actionable data that enables businesses to optimize their operations. Used together, these tools can identify patterns indicating an imminent failure. In the bearing example, the data could be used to identify the pattern causing the bearing temperature to rise beyond its normal operating range. Once implemented, this approach can significantly increase productivity and reduce maintenance costs.
prev
next
Main AVEVA Predictive maintenance features
Integrate your own algorithm
Data scientists can incorporate their own algorithms into the predictive maintenance loop using Python or similar languages. Monitor all analytics within one application and enhance custom algorithms with built-in model templates, data cleansing, alerting, fault diagnostics, prescriptive actions, forecasting, and a case library. Leverage real-time and historical data through seamless integration with AVEVA software.
Anomaly Detection
Receive advanced alert notifications and utilize case management for knowledge capture and comprehensive reporting. Users without programming or data science knowledge can deploy, validate, and interpret predictive model results. The software’s predefined templates accelerate configuration, deployment, and scaling.
Fault Diagnostics
Leverage customized data and diagnostic tools for precise real-time insights, enabling timely and consistent analysis of alert conditions for quick problem diagnosis and remediation. Assess how current performance aligns with asset fault conditions and identify individual sensors contributing to faults. Employ sensor preprocessing to detect problems and inconsistencies, thereby improving data quality.
Monitor and compare
Easily monitor abnormal conditions during transient periods, such as start-ups and shutdowns. Automatically identify and compare previous transient events using data from the historian.
Which AVEVA software meets your predictive maintenance needs?
AVEVA Predictive Analytics
Reduce unexpected downtime and enhance asset dependability through self-service analytics.
Data Analytics & AI
AVEVA PI Data Infrastructure
Hybrid solution ready to manage your operational data, from the Edge to the Cloud.
Data Analytics & AI
prev
next
Take your data analytics and AI strategy further.
Reduce greenhouse gas emissions and operational costs. Comply with stringent environmental regulations and meet sustainability goals. Discover strategies for carbon footprint reduction, eco-friendly practices, green technology, and improving corporate social responsibility (CSR). Prioritize sustainability and energy efficiency.
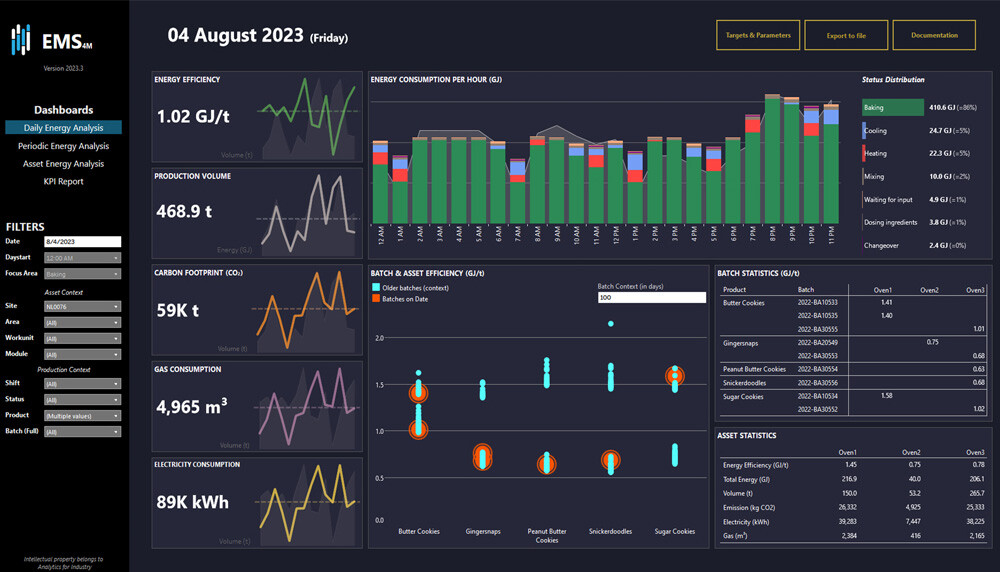
Data analytics & AI: Latest News
prev
next
prev
next